Retrieval Augmented Generation (RAG) works as a backbone for interacting with an enterprise’s own data via Conversational Question Answering (ConvQA). In a RAG system, a retriever fetches passages from a collection in response to a question, which are then included in the prompt of a large language model (LLM) for generating a natural language (NL) answer.
Over the last four years, RAG has been a topic of intense investigation. We posit that despite several advanced features, past works suffer from two basic concerns, one at each end of the pipeline: (i) at the beginning, documents are typically split into chunks (usually one or more passages) that are indexed on their content. When these chunks are retrieved and fed to an LLM, they often lack supporting context from the document, which adversely affects both retrieval, and subsequent answering; and (ii) at the end, attribution mechanisms that attach provenance likelihoods of the answer to the retrieved units of evidence, are solely based on statistical similarity between the answer and the evidence: these are not causal, but rather only plausible explanations.
We at the NLP team at Fraunhofer IIS, demonstrate RAGONITE (overview below), a RAG system that remedies the above concerns by: (i) contextualizing evidence with source metadata and surrounding text; and (ii) computing counterfactual attribution, a causal explanation approach where the contribution of an evidence to an answer is determined by the similarity of the original response to the answer obtained by removing that evidence. To evaluate our proposals, we release a new benchmark ConfQuestions: it has 300 hand-created conversational questions, each in English and German, coupled with ground truth URLs, completed questions, and answers from 215 public Confluence pages. These documents are typical of enterprise wiki spaces with heterogeneous elements. Experiments with RAGONITE on ConfQuestions show the viability of our ideas: contextualization improves RAG performance, and counterfactual explanations outperform standard attribution.
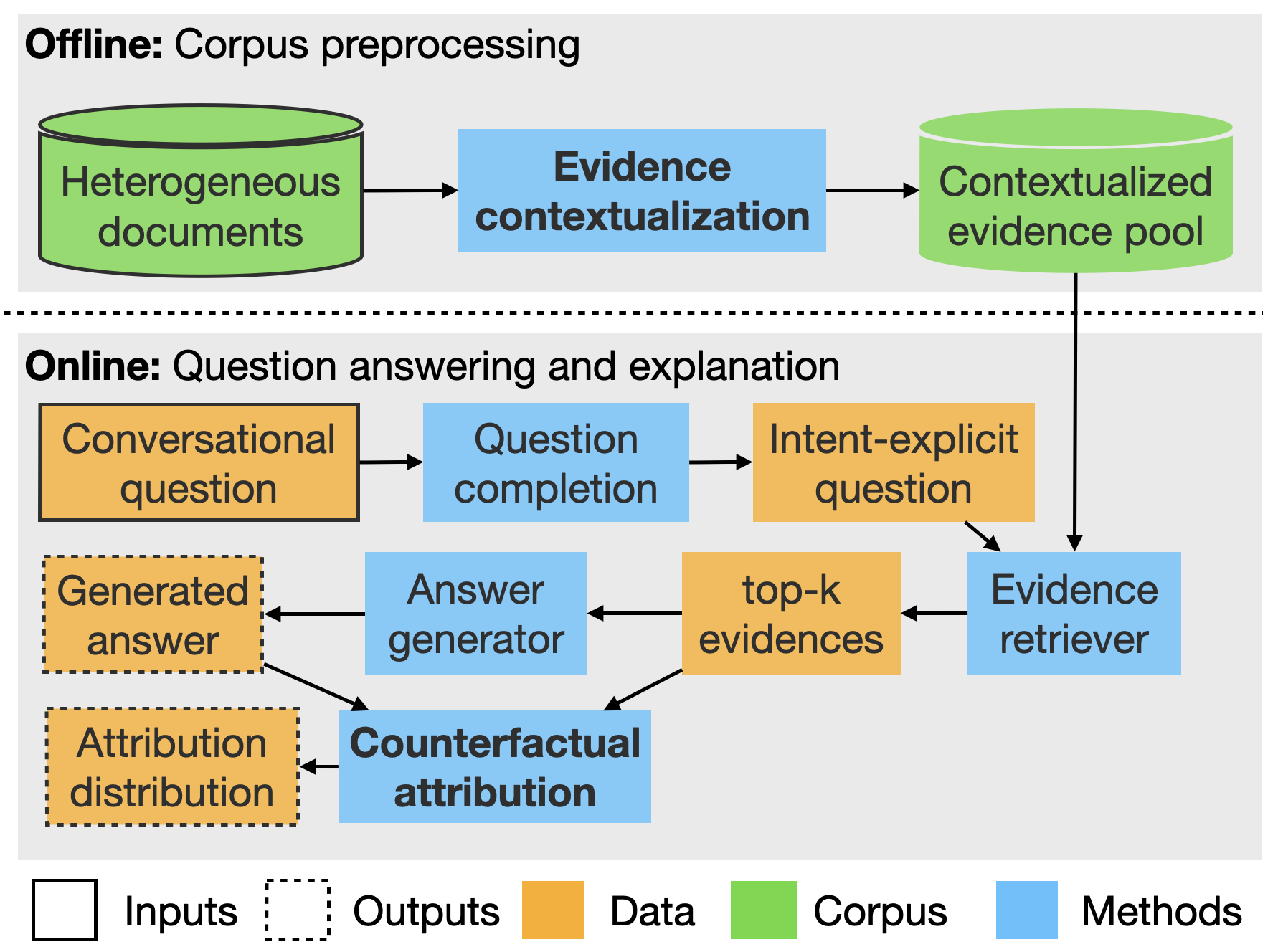
RAGonite is an explainable RAG pipeline, which makes every intermediate step open to scrutiny by an end user. We propose flexible strategies for adding context to heterogeneous evidence, and causally explain LLM answers using counterfactual attribution. For more information, please visit our public GitHub repository at https://github.com/Fraunhofer-IIS/RAGonite.
Author: Rishiraj Saha Roy, Joel Schlotthauer, Chris Hinze, Andreas Foltyn, Luzian Hahn, and Fabian Küch